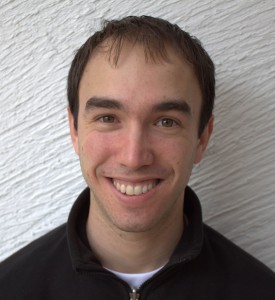
David Sontag, PhD
Assistant Professor of Computer Science and Data Science
New York University
http://cs.nyu.edu/~dsontag/
http://clinicalml.org/
David Sontag is an Assistant Professor of Computer Science and Data Science at New York University’s Courant Institute. His research focuses on using machine learning to improve health care, including development of novel algorithms for early detection of Type 2 diabetes, modeling disease progression, electronic phenotyping, visualization, and clinical decision support in emergency departments. David has written over 30 publications, three receiving best paper awards. Prior to joining NYU, he was a postdoctoral researcher at Microsoft Research. David received a B.A. from the University of California, Berkeley, where he was awarded the highest recognition in the Electrical Engineering & Computer Science department and was a University Medal Finalist. He also received a M.S. and Ph.D. from the Massachusetts Institute of Technology, receiving the Sprowls Award for the best doctoral thesis in Computer Science. In 2014, David was recognized with the National Science Foundation CAREER Award.
Y. Halpern, Y.D. Choi, S. Horng, D. Sontag. Using Anchors to Estimate Clinical State without Labeled Data. American Medical Informatics Association (AMIA) Annual Symposium, Nov. 2014.
X. Wang, D. Sontag, F. Wang. Unsupervised Learning of Disease Progression Models. ACM SIGKDD Conference on Knowledge Discovery and Data Mining (KDD), Aug. 2014.
Y. Jernite, Y. Halpern, S. Horng, D. Sontag. Predicting Chief Complaints at Triage Time in the Emergency Department. NIPS 2013 Workshop on Machine Learning for Clinical Data Analysis and Healthcare, Dec. 2013.
Y. Halpern, D. Sontag. Unsupervised Learning of Noisy-Or Bayesian Networks. Uncertainty in Artificial Intelligence (UAI) 29, July 2013.
S. Arora, R. Ge, Y. Halpern, D. Mimno, A. Moitra, D. Sontag, Y. Wu, M. Zhu. A Practical Algorithm for Topic Modeling with Provable Guarantees. 30th International Conference on Machine Learning (ICML), 2013.
Y. Halpern, S. Horng, L. A. Nathanson, N. I. Shapiro, D. Sontag. A Comparison of Dimensionality Reduction Techniques for Unstructured Clinical Text. ICML 2012 Workshop on Clinical Data Analysis, July 2012.
T. Koo, A. Rush, M. Collins, T. Jaakkola, and D. Sontag. Dual Decomposition for Parsing with Non-Projective Head Automata. Empirical Methods in Natural Language Processing (EMNLP), 2010.
T. Jaakkola, D. Sontag, A. Globerson, M. Meila. Learning Bayesian Network Structure using LP Relaxations. 13th International Conference on Artificial Intelligence and Statistics (AI-STATS), 2010.
D. Sontag, T. Meltzer, A. Globerson, Y. Weiss, T. Jaakkola. Tightening LP Relaxations for MAP using Message Passing. Uncertainty in Artificial Intelligence (UAI) 24, July 2008.
B. Milch, B. Marthi, S. Russell, D. Sontag, D. L. Ong, and A. Kolobov. BLOG: Probabilistic Models with Unknown Objects. Proc. 19th International Joint Conference on Artificial Intelligence (IJCAI):1352-1359, 2005.